Understanding AI Content Generation: How It Works
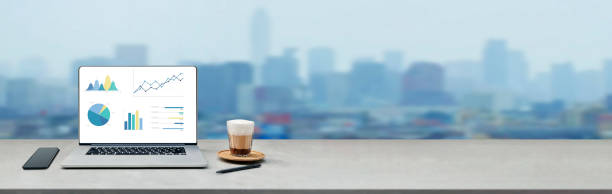
AI content generation is a fascinating field that leverages advanced algorithms and machine learning to produce text, images, and other forms of media. At its core, this technology involves the use of models trained on vast amounts of data to understand patterns in language and content creation. Here’s an overview of how it works, the underlying technologies, and its applications.
The Foundations of AI Content Generation
AI content generation primarily relies on natural language processing (NLP), a branch of artificial intelligence focused on the interaction between computers and human language. NLP enables machines to comprehend, interpret, and generate human language in a way that is both meaningful and contextually relevant.
The most common method of AI content generation utilizes deep learning, particularly through models known as transformers. These models, such as OpenAI's GPT (Generative Pre-trained Transformer), are designed to predict the next word in a sentence based on the words that came before it. By training on diverse datasets, these models learn various aspects of language, including grammar, context, and even stylistic nuances.
The Training Process
Training an AI model involves exposing it to vast datasets containing examples of text from books, articles, websites, and other sources. This dataset acts as a foundation for the model to learn from, allowing it to recognize patterns and associations in language. The training process consists of two main phases:
Pre-training: In this phase, the model learns to predict the next word in a sentence. By doing this repeatedly on a massive scale, it develops a general understanding of language structure and context.
Fine-tuning: After pre-training, the model undergoes fine-tuning on more specific datasets, often tailored to particular applications or industries. This helps the AI generate content that is more relevant to specific contexts, such as marketing, technical writing, or creative storytelling.
Content Generation Techniques
Once trained, AI can generate content in several ways:
Text Completion: The AI can take a prompt and generate text that continues the narrative, providing coherent and contextually appropriate responses.
Summarization: AI can condense longer texts into concise summaries, retaining the essential information while eliminating unnecessary details.
Translation: AI models can translate text from one language to another, maintaining the meaning and tone of the original content.
Creative Writing: AI can also produce poetry, short stories, and other forms of creative writing by understanding themes, styles, and emotions.
Applications of AI Content Generation
The applications of AI content generation are vast and varied. In marketing, businesses utilize AI to create engaging ad copy, product descriptions, and social media posts, helping them save time and resources. In journalism, AI can generate news reports based on data and trends, allowing for quicker dissemination of information. Additionally, in education, AI can provide personalized learning experiences, creating tailored content based on individual student needs.
Ethical Considerations
While AI content generation offers numerous benefits, it also raises ethical questions. Concerns about misinformation, plagiarism, and the potential for biased content are significant. As AI continues to evolve, ensuring responsible use and implementation will be crucial for maintaining trust and integrity in generated content.
Conclusion
AI content generation is transforming how we produce and consume information. By harnessing the power of advanced algorithms and vast datasets, AI can create content that is both engaging and relevant. As technology progresses, it will be essential to navigate the challenges and opportunities that come with it, ensuring that AI remains a beneficial tool for creators and consumers alike.